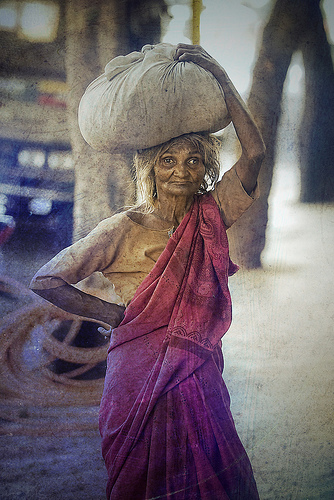
Sticking to the old, proven concepts have often turned out to be a good strategy in orthopaedics. Switching hip implants can be both cumbersome and hazardous. The image is CC by Trey Ratcliff.
In my research I focus on patient factors and their impact on re-operation rates after total hip arthroplasties. While they do matter, the implant itself seems to be even more important. One of the most successful implant in the Swedish Hip Arthroplasty Registry is the Link Lubinus SP II implant, while some may argue that it’s an awesome design, I would argue that the tools that it comes with are awesome, thus limiting surgeon’s mistakes. This importance of skill is nicely illustrated in Peltola et al’s recent study, where they found an increased risk for revision during the first 3 years when introducing new implants, hazard ratio was 1.3 (95% CI 1.1 to 1.5) for the first 15 arthroplasties. This increased risk early on is commonly referred to as the learning curve, and may vary between implants.
Peltola et al used the Finnish arthroplasty register, looked for how many stems/cups had been implanted of that kind at the particular hospital, categorized into five groups (1-15, 16-30, 31-50, 51-100, and > 100), and then looked at early and late revision rates. The only group with statistical significance was the 1-15, there were some other groupings but after reading the table 5 a couple of times I’m not sure it actually added anything but confusion. Although the other groups were not significant, this is clearly clinically relevant in Finland as one in seven patients receive an implant during its introduction.
I’m glad to see that more advanced statistical approaches are gaining ground, and their methods section was extensive. Even though I’m left with a few thoughts:
- Introducing new implants at large volume hospitals, assuming that these have more surgeons, the time until each surgeon becomes experienced should increase, i.e. an interaction between hospital volume and the number of implanted stems/cups of the new kind.
- While categories often are used, it would be interesting to see a more dynamic interpretation of the number of surgeries – as a spline enthusiast I would suggest applying these. It is interesting that they didn’t do this, while they at the same time used a smoother for the revision risk, se below image.
A smoothing plot showing the hazard in relation to the years after surgery, early on there is an increased risk that then drops to a minimum around 4-5 years, where after it again increases. As a technical note it is interesting that they forgot to add the y-axis label, “Smoothed hazard function” is not a valid y-axis label in my opinion.
- Were some of these new implants early on abandoned? It is plausible that a clinic tries a certain implant, finds it clumsy or underperforming, and abandons it early on. This would introduce a negative bias for the small series.
- Sometimes an implant can boomerang, i.e. come and go, unclear if this taken into account.
- They adjusted for sex and age, and table 3 indicates little difference. Shouldn’t the adjustment rather be implant fixation type or some other implant confounder? It would also be interesting to see if there is a difference in introduction estimate depending on what kind of fixation is used. Uncemented stems are for instance more susceptible to fractures and surgeon’s experience is critical, while cementing may in the inexperienced hand both lead to malpositioning and a poor fixation due to correction while the cement hardens.
- Table 5 indicates the most common implants and the implants learning curve differ, annoyingly they chose to number the implants putting unnecessary effort on the reader. This could be used for the above bullet but the wide confidence intervals suggest that this grouping is not optimal for the data set.
- I’m also hesitant about the exclusion criteria, why did they include bone grafts – this is clearly a rare procedure that may demand very specific implants. They should also have excluded implants with small series, a lot of implants are tested in small research series – if these are excluded small series will naturally have a negative bias.
As a surgeon I’m overall pleased to see these results – my expertise matters! Patients sometimes ask me what implant we use, I always answer: one that has a proven track record and that I’m familiar with. While the study may seem complicated if you look at the method section, the conclusion is simple: don’t change implant on a whim – make sure it will truly be better than your old one or you’re putting patients at risk.